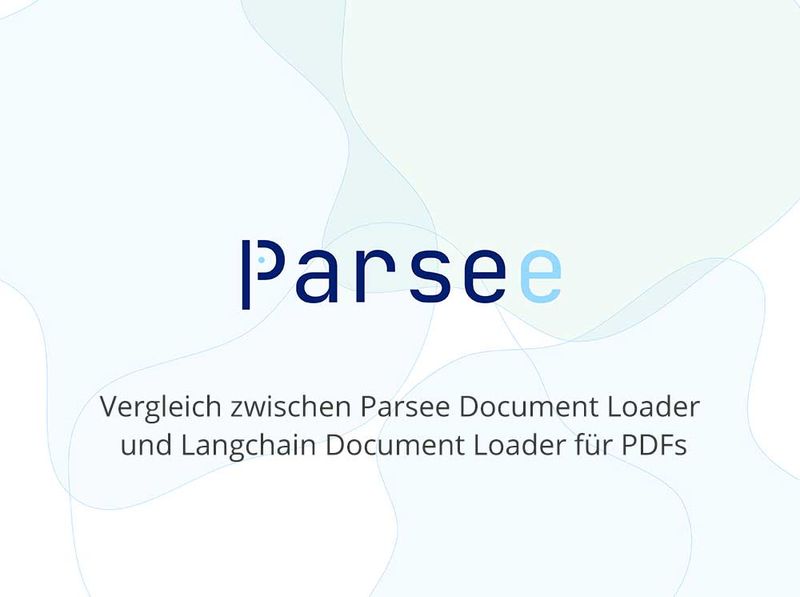
Open Source Framework für Datenextraktion und -Strukturierung
Revolutionieren Sie die Datenextraktion mit Parsee.ai: Nutzen Sie LLMs und benutzerdefinierte KI-Modelle, um PDFs, HTMLs und Bilder in vollständig strukturierte Daten zu verwandeln.

Wesentliche Merkmale
Parsee stellt ein einfaches Framework für die Strukturierung von Daten aus den gängigsten unstrukturierten Datenquellen bereit.
Sie können sofort mit der Strukturierung von Daten beginnen, indem Sie LLMs verwenden, die als "universelle" Modelle dienen können. Sobald Sie einige Daten extrahiert haben, können Sie Datensätze erstellen und benutzerdefinierte, aufgabenspezifische Modelle trainieren, die LLMs in Bezug auf Genauigkeit und Kosteneffizienz übertreffen können.
Parsee Cloud
Beispiel für Extraktionsvorlagen
Erstellen Sie mühelos typsichere Extraktionsvorlagen und speichern Sie diese in der Parsee Cloud. Alternativ können Sie die Vorlagen auch im visuellen Editor der Parsee Cloud erstellen und lokal laden.
# Define questions in free text form
question = "What is the invoice total?"
# Define output types
output_type = OutputType.NUMERIC
# Define meta items: information that is associated with the main question we are asking, such as time periods, currencies, units etc.
meta_currency = "What is the currency?"
meta_currency_output_type = OutputType.LIST
meta_item = MetaItem(meta_currency_question, meta_currency_output_type, list_values=["USD", "EUR", "Other"])
invoice_total = StructuringItem(question_to_be_answered, output_type, meta_info=[meta_item])
job_template = create_template([invoice_total])
# Optional: save template to Parsee Cloud
cloud = ParseeCloud("YOUR_KEY")
template_id = cloud.save_template(job_template)
# Or load any template from Parsee Cloud (templates are shareable in your organisation)
template = cloud.get_template(template_id)
Dokumente laden und Aufträge lokal oder in der Parsee Cloud ausführen
Extrahieren Sie strukturierte Daten einfach mit dem Parsee Document Loader und einer großen Auswahl an vordefinierten Modellen:
# Use default loader to determine the document type automatically:
document = load_document("../tests/fixtures/Midjourney_Invoice-DBD682ED-0005.pdf")
# you can also build a custom document converter of course if needed for your use-case
# define a model, here you can use all open source models from Replicate for example: https://replicate.com/
replicate_api_key = os.getenv("REPLICATE_KEY")
replicate_model = replicate_config(replicate_api_key, "mistralai/mixtral-8x7b-instruct-v0.1")
# run the extraction using an extraction template (see steps above)
_, _, answers = run_job_with_single_model(document, job_template, replicate_model)
answers[0].class_value
>> 11.9
answers[0].meta[0].class_value
>> 'USD'